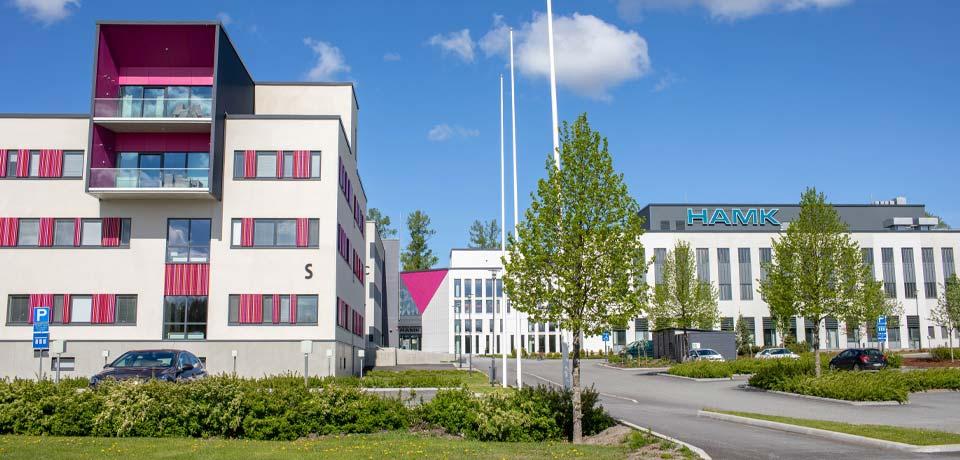
Wangkang Jin & Jukka Pulkkinen
The energy system consists of providers and consumers, and traditionally consumers are not actively engaged in optimizing the overall system to minimize energy consumption. One challenge for the energy system is peak consumption, when a provider may need to start fossil fuel-fired power plants to generate enough energy. This is very costly and leads to a higher CO2 footprint. Therefore, one development topic is to engage consumers to reduce their energy consumption at peak periods to avoid the unnecessary start-up of costly and polluting energy production. Our study aims to simulate whether HAMK’s S-building in Hämeenlinna University Centre can be used as a flexible energy consumer to reduce district heat demand in peak periods while keeping indoor temperatures at accepted tolerances. The simulation results are promising and indicate that quite significant energy reductions can be made without a major impact on indoor temperatures. This study was conducted as part of the ÄlyKaupunki project, which receives funding from the European Regional Development Fund (Euroopan Aluekehitysrahasto).
Introduction
Buildings offer good short-term heat storage capacity to improve energy efficiency in district heating systems. The key idea behind this is to cut peak loads in the system by reducing energy consumption in the selected buildings through the use of their thermal mass (Hietaharju, 2021). This means that reducing energy consumption will have an impact on indoor temperature after certain delays, and this impact can be very minor in brief energy consumption reductions. This requires systematic co-operation between energy suppliers and consumers. In practice, the challenge is that the consumers are not engaged in the optimization of the overall energy system (Fujii et al., 2022). A data-driven approach to modelling the energy system in a way that consumers can be used in demand response has been an important research topic in the literature (Pritoni et al., 2021).
In our study, we have analyzed whether HAMK’s S-building in Hämeenlinna University Centre can be used in demand response in the way that district heating demand can be adjusted and at the same time we can ensure sufficient indoor temperature. Our study aims to find answers to the following research questions:
- Modeling of the district heating system: Can a correlation between district heat demand and indoor temperature in the building be found?
- Can we use this model to adjust district heating demand in the building so that the indoor temperature stays within tolerances?
Methodology
One important element to creating demand response capability is to have storage for the energy. The short-term storage of electricity is already in commercial use and more practical applications are becoming available. District heating plays an important role in the Finnish energy system; it is particularly widely used in the heating of buildings (Ministry of the Environment, 2020). Buildings offer great potential to act as short-term storage for heat, but they are not yet used for this purpose in practice (Kontu, 2020). In his academic dissertation, Hietaharju (2021) presented a method to model buildings so that they can be used for heat storage in the energy system. In our study, we apply his approach to model the dynamic behavior of the S-building in Hämeenlinna University Centre and to simulate different scenarios to use the building for heat storage.
The indoor temperature model is derived from the academic dissertation (Hietaharju, 2021):
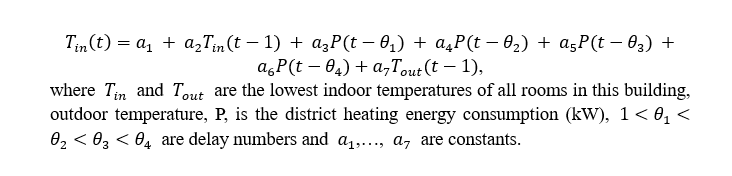
Firstly, the best delays with the minimum root mean square error (RMSE) need to be tuned. Based on these delays, an indoor temperate prediction model is built.
Hietaharju (2021) also presents a method to calculate the model parameters based on the physical features of the building. However, we chose to use a data-driven approach to calculate the model parameters, because this would also offer better opportunities to implement the solution in industrial applications. A data-driven approach is a cost-effective method compared to physical modelling, which is very time-consuming in practice. On the other hand, a data-driven method can be automated by using the collected data and algorithms and therefore personalization is easier to implement in real, large-scale applications. It is also worth mentioning that the only necessary measurements are indoor and outdoor temperature, which are very easy to implement. Typically, the indoor temperature is measured by a building automation system; if not, it is very easy to measure using a separate measurement device.
In the literature, several studies were conducted using artificial neural networks (ANN) to model the heating systems in different buildings (Huang et al., 2015; Pritoni et al., 2021; Mathews et al., 2001). Nevertheless, in our study, we use the linear model as it has some practical advantages, such as easy modelling and implementation without the need for so much data for training, and less computer calculation capacity is needed. On the other hand, the linear model naturally cannot model nonlinear features as ANN can.
The developed model was used to test whether the S-building can be used for heat storage. Two scenarios were simulated by reducing district heating:
- The first scenario was to simulate how long, starting at 7:00, energy could be reduced by 70% while the temperature in all the building’s rooms remained above 20℃.
- The second scenario was to simulate how long the heating energy can be reduced if it is cut by 70% at 18:00 and the lowest indoor temperature of the building is required to reach 20℃ by 8:00 the next morning.
The first scenario would provide a solution for the district heating provider in cases where the morning represents a peak in consumption, and they would like to reduce demand to stabilize the overall heat demand. It is typical for heat demand to peak in the morning due to several reasons. This is very important if they need to start more costly energy production to respond to the peak demand. The second scenario aims to reduce the overall heat demand because typically many buildings are not used in the evening and at night they could easily reduce the temperature during this period.
The developed indoor temperature model is as follows:
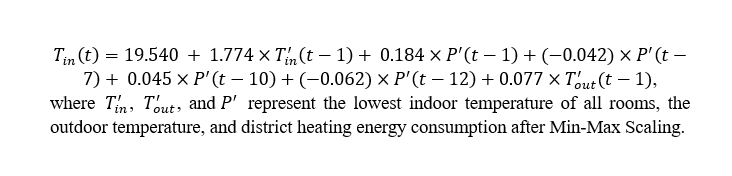
The data was collected between 01.13.2022 and 03.01.2022. Eighty per cent of the data (from 01.13.2022 to 02.19.2022) was used for training and 20% for testing (from 02.19.2022 to 03.01.2022).
The model results in the key performance indicators listed in Table 1. The mean absolute percentage error (MAPE) was 0.54% for the training and 1.01% for the testing datasets. Modelling results are presented in Figure 1, where the indoor air temperature is predicted for 4, 12, and 48 hours. We can see that the measured temperature is very close to the simulations. This means that we can predict the temperature accurately by using the outside temperature and district heating demand.
Algorithm | Dataset | MSE ((C)²) | RMSE (C) | MAE (C) | MAPE |
Linear model | Training | 0.032 | 0.178 | 0.111 | 0.54% |
Linear model | Testing | 0.093 | 0.304 | 0.210 | 1.01% |
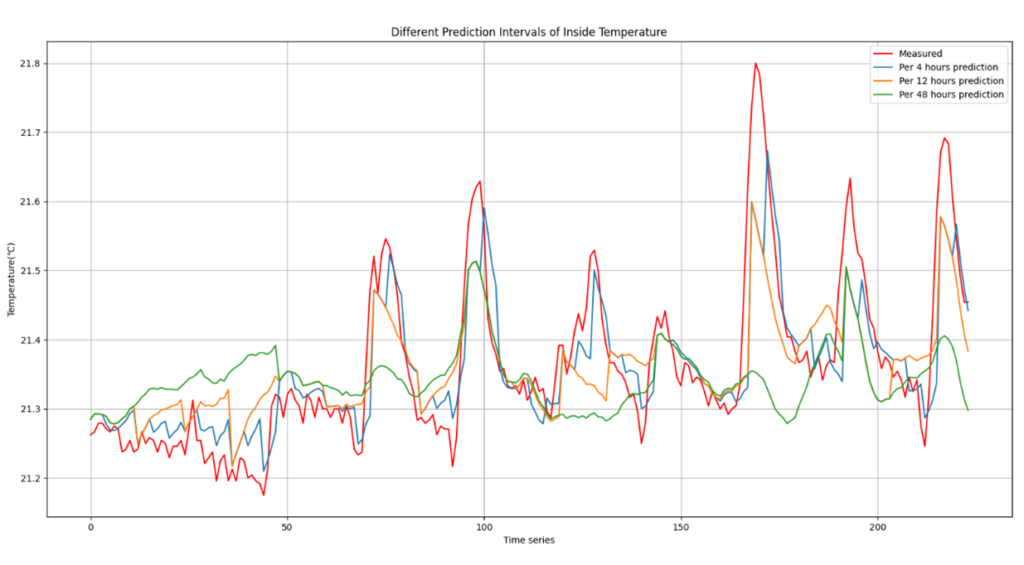
The results for the scenarios are shown in Figure 2 and Figure 3. For scenario 1, the longest period is from 7:00 to 12:00 if the temperature of all rooms is required to stay above 20℃ during this period. For scenario 2, heating could be reduced by 70% from 18:00 to 5:00 the next morning if the indoor temperature is required to get back to 20℃ the next morning. The results indicate that our building has good heating storage capacity, which shows huge potential for the demand response to increasing energy efficiency and system flexibility.
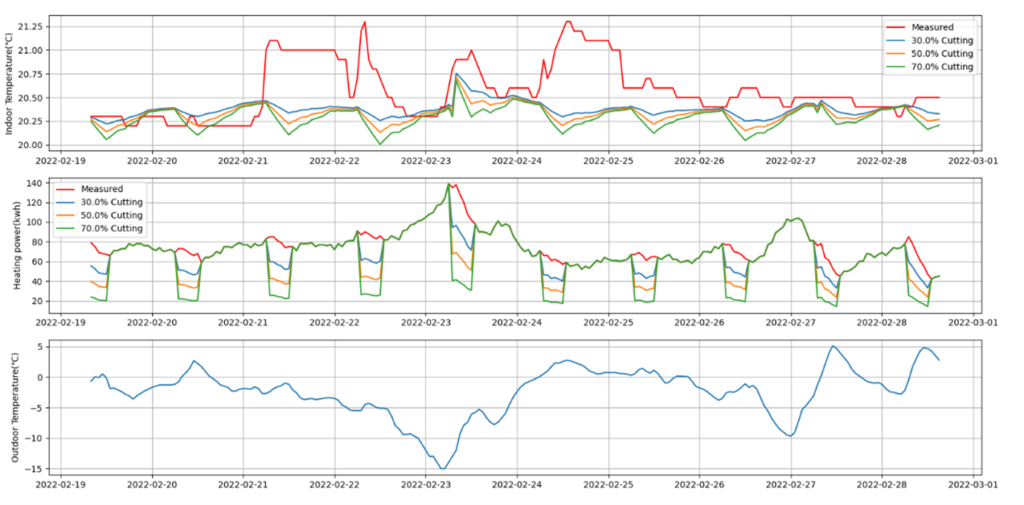
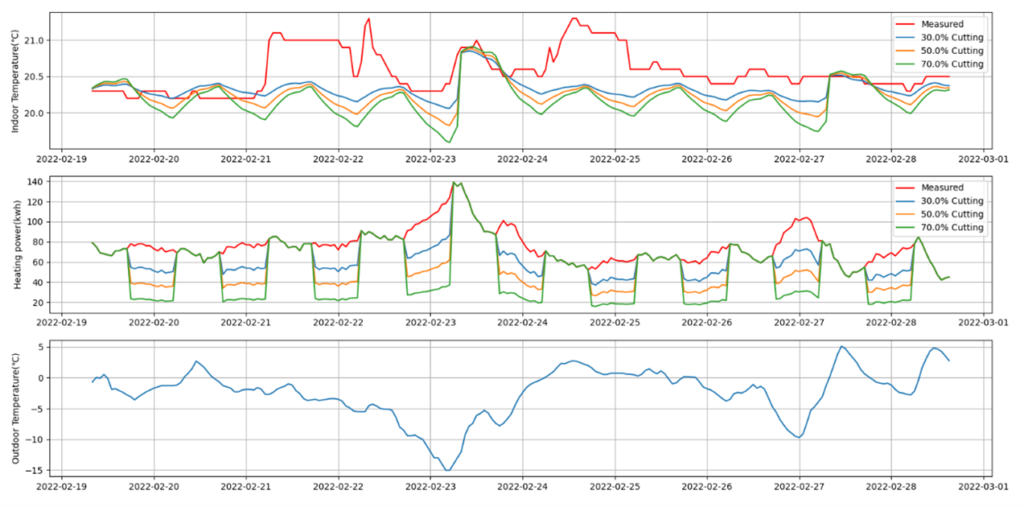
Conclusions
We have analyzed the possibility of using HAMK’s S-building as heat storage to create demand response capability. The correlation between district heat demand and indoor temperature was developed with the use of the method presented in an academic dissertation (Hietaharju, 2021). The developed model offers good key performance indicators and therefore it is a good basis for simulating heat storage capability. Two different scenarios effectively demonstrate the capability, and ultimately the indoor temperature stays well within the accepted tolerances, even if the district heat demand is reduced by 70% for several hours.
More research is needed to create a new industrial application for demand response. These simulation results should be evaluated against real tests in the real environment. The algorithm would be easy to develop to the next level for it to calculate the optimum energy reduction and time frame to reach minimum energy consumption.
The industrial applications would represent new types of business models, where building owners can offer their buildings to be used as heat storage. This would require development in co-operation between different parties, including district heat providers, building owners, and optimization companies.
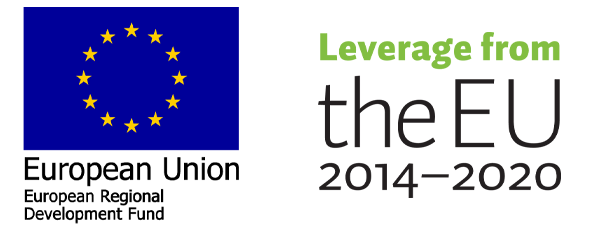
Authors
Wangkang Jin is studying currently autonomous systems in KTH Royal Institute of Technology, Sweden and before starting in KTH he completed several machine learning related courses in Aalto university. Now he is working in HAMK Smart research unit as project engineer in ÄlyKaupunki project.
Dr. Jukka Pulkkinen holds a Ph.D. in Automation and System Technology from the Helsinki University of Technology. Currently, he is the director of HAMK Smart research unit at Häme University of Applied Sciences. From 2003 to 2017, he held several management positions in Valmet. He has also held a few research positions at Tampere University of Technology in the 1990s. His current research interests include business strategy and management, operation research, as well as digital services.
References
Fujii, T. Y., Hayashi, V. T., Arakaki, R., Ruggiero, W. V., Bulla, R., Hayashi, F. H., & Khalil, K. A. (2022). A Digital Twin Architecture Model Applied with MLOps Techniques to Improve Short-Term Energy Consumption Prediction. Machines, 10(1). https://doi.org/10.3390/machines10010023
Hietaharju, P. (2021). Predictive optimization of heat demand utilizing heat storage capacity of buildings. (Acta Universitatis Ouluensis. C, Technica) [Dissertation, The University of Oulu]. Jultika. http://urn.fi/urn:isbn:9789526231310
Huang, H., Chen, L., & Hu, E. (2015). A neural network-based multi-zone modelling approach for predictive control system design in commercial buildings. Energy and Buildings, 97, 86–97. https://doi.org/10.1016/j.enbuild.2015.03.045
Kontu, K. (2020). Customer-centred development paths for district heating industry. (Aalto University publication series DOCTORAL DISSERTATIONS, 6/2020) [Dissertation, Aalto University]. Aaltodoc. http://urn.fi/URN:ISBN:978-952-60-8910-2
Mathews, E. H., Botha, C. P., Arndt, D. C., & Malan, A. (2001). HVAC control strategies to enhance comfort and minimise energy usage. Energy and Buildings, 33(8), 853–863. https://doi.org/10.1016/S0378-7788(01)00075-5
Ministry of the Environment. (2020). Pitkän aikavälin korjausrakentamisen strategia 2020–2050. https://ym.fi/korjausrakentamisen-strategia
Pritoni, M., Paine, D., Fierro, G., Mosiman, C., Poplawski, M., Saha, A., Bender, J., & Granderson, J. (2021). Metadata schemas and ontologies for building energy applications: A critical review and use case analysis. Energies, 14(7), 1–37. https://doi.org/10.3390/en14072024